8th April 2022, Dr Chee L Khoo
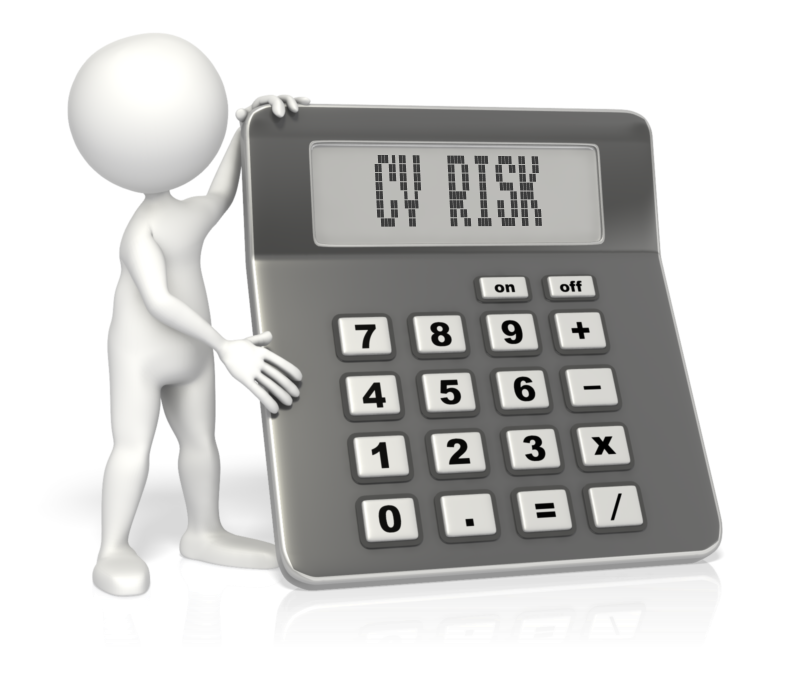
CVD is the major cause of mortality and morbidity in patients with type 2 diabetes (T2D). There are many hundreds of cardiovascular risk prediction tools around. Well, whenever you have many versions of a particular tool, it usually tells us that none of them are particularly good in what they are meant to do – that is to predict the risk of a cardiovascular event. Many of these tools are not validated in patients with diabetes. Some just automatically deem all patients with diabetes as “high risk” without considering their HbA1c or the duration of their disease. That is not helpful for us in clinical practice. How well do these tools predict in patients with T2D?
Further, most of the tools looked at atherosclerotic cardiovascular disease (coronary artery disease and strokes) and not heart failure (HF), atrial fibrillation (AF) or peripheral artery disease (PAD) which are more prevalent in patients with T2D. Some prediction tool use a broader definition of major cardiovascular outcomes (CVD+) which include HF and AF.
How accurate are the cardiovascular prediction tools?
In a recent review Dziopa K et al used data extracted from three English EHR sources: primary care records from the Clinical Practice Research Datalink (CPRD), Hospital Episodes Statistics (HES) and national death registration from the Office for National Statistics (ONS) to test 22 prediction tools (1). They obtained a contemporary, representative sample of 168,871 UK-based individuals with type 2 diabetes (age ≥18 years without pre-existing CVD+).
Individuals were followed up from their initial T2D diagnosis until their first cardiovascular event, death, end of study (5 February 2018) or 10 year follow-up landmark whichever occurred first. Individuals with previous episodes of AF or other CVD were excluded. A CVD event was defined as the first occurrence of fatal or non-fatal myocardial infarction (MI), sudden cardiac death, ischaemic heart disease, fatal or non-fatal stroke, or PAD since diagnosis of type 2 diabetes. They also defined CVD+ as CVD including HF and/or AF. They then used CVD risk prediction tools to test to see how accurate and useful they were in this cohort of patients.
Out of the 22 identified CVD risk prediction models, 9 were derived in individuals with type 2 diabetes alone:
- Risk Equations for Complications Of type 2 Diabetes [RECODE] [2]
- Diabetes Audit and Research in Tayside [DARTS] [3]
- UKPDS 56 [4]
- UKPDS 68 C-HF [5]
- UKPODS Stroke [5]
- UKPDS 82 CHD [6]
- UKPDS 82 C-HF [6]
- CHS Basic [7]
- CHS Advanced [7])
13 scores enrolled both non-diabetic individuals and individuals with type 2 diabetes:
- SCORE CVD [8]
- SCORE CHD [8]
- Finrisk Stroke [9]
- Finrisk CHD [9]
- Finrisk CVD [9]
- Framingham 1991 CHD [10]
- Framingham 1991 CVD [10]
- Framingham 1991 Stroke [10];
- Framingham 1998 [11];
- QRISK2 [12];
- QRISK3 [13];
- ASCVD [14]
- Reynolds Risk [15, 16]),
The Evaluation
The prediction tools were evaluated on their ability to predict a CVD event using C statistic. The C statistic varies from 1.0 (perfect discrimination) to 0.5 (random chance). It has been suggested that a C statistic below 0.70 indicates inadequate discrimination, between 0.70 and 0.80 acceptable discrimination, and between 0.80 and 0.90 excellent model discrimination.
They then used the data to recalibrate the various tools on a small sample of the data (10%). Once calibrated, the tools were re-used in the prediction algorithm.
Results
During a median follow-up time of 10 years since T2D diagnosis, 38,335 (22.70%) individuals suffered a CVD, AF or HF event. Of these, 29,025 (17.19%) had a CVD event, 20,628 (12.22%) CHD, 13,826 (8.19%) AF, 9465 (5.6%) HF and 6727 (3.98%) stroke.
What did they find?
Most prediction scores almost uniformly underestimated the risk of CVD+ (CS: from 0.41 to 0.88) the exceptions being the Framingham 1991 CVD and DARTS scores which systematically overestimated risk. The scores were even worse in individuals with diabetes and established CVD at baseline (C statistics close to 0.50).
Prediction scores with more than 10 predictors did not necessarily outperform scores with fewer variables: For example QRISK3 (19 variables) for CVD+ had a C statistic of 0.68, compared with a C statistic of 0.69 for SCORE CVD (6 variables) and a C statistic of 0.69 for the Framingham1998 score (7 variables). Similar results were obtained for the CVD only outcome.
The scores derived from individuals with diabetes did not outperform scores derived in a population of non-diabetic individuals. Diabetes specific scores did not appear to be superior to scores derived for the general population, and in fact were outperformed by, for example, the general population SCORE CVD rule. Scores with many additional features did not outperform those with fewer and more readily available (in primary care) predictors.
Simple recalibration markedly improved score performance, repurposing scores intended to predict any CVD or CHD to accurately predict stroke, AF and HF risk The predictive performance of the risk scores was markedly poorer in individuals with pre-existing CVD at the time of T2D diagnosis (C statistic ranged from 0.50 to 0.54 for the CVD outcome). Remember, most of the prediction models were developed in individuals without clinical manifestations of CVD and were not validated in people with established CVD. We don’t have data on how the tools work in patients with pre-exisiting CVD. We did not observe a general benefit of diabetes-specific rules (including diabetes-specific variables) compared with scores derived in samples with a mixture of individuals with type 2 diabetes and the general population.
Despite comparing risk prediction tools derived over the course of more than three decades, during which health and healthcare have generally improved, the almost uniform performance of these scores in the present contemporary sample of individuals with type 2 diabetes illustrates that these healthcare changes have not affected the external performance of these models.
In summary, this review illustrate that current CV prediction tools derived from the general population performed poorly and performed even worse in people with type 2 diabetes. However, CVD risk scores derived in individuals with diabetes did not in general perform better, emphasising the difficulties of accurately predicting CVD in a relatively high-risk population. With some recalibration, they may perform better.
References:
1. Dziopa K, Asselbergs FW, Gratton J, Chaturvedi N, Schmidt AF. Cardiovascular risk prediction in type 2 diabetes: a comparison of 22 risk scores in primary care settings. Diabetologia. 2022 Apr;65(4):644-656. doi: 10.1007/s00125-021-05640-y
2. Basu S, Sussman JB, Berkowitz SA, Hayward RA, Yudkin JS (2017) Development and validation of risk equations for complications of type 2 diabetes (RECODe) using individual participant data from randomised trials. Lancet Diabetes Endocrinol 5(10):788–798. https://doi.org/10.1016/S2213-8587(17)30221-8
23. Donnan PT, Donnelly L, New JP, Morris AD (2006) Derivation and validation of a prediction score formajor coronary heart disease events in a U.K. type 2 diabetic population. Diabetes Care 29(6): 1231–1236. https://doi.org/10.2337/dc05-1911
4. Stevens RJ, Kothari V, Adler AI, Stratton IM, United Kingdom Prospective Diabetes Study (UKPDS) Group (2001) The UKPDS risk engine: a model for the risk of coronary heart disease in type II diabetes (UKPDS 56). Clin Sci Lond Engl 1979 101(6):671–679
5. Clarke PM, Gray AM, Briggs A et al (2004) A model to estimate the lifetime health outcomes of patients with type 2 diabetes: the United Kingdom prospective diabetes study (UKPDS) outcomes model (UKPDS no. 68). Diabetologia 47(10):1747–1759. https://doi.org/10.1007/s00125-004-1527-z
6. Hayes AJ, Leal J, Gray AM, Holman RR, Clarke PM (2013) UKPDS outcomes model 2: a new version of a model to simulate lifetime health outcomes of patients with type 2 diabetes mellitus using data from the 30 year United Kingdom prospective diabetes study: UKPDS 82. Diabetologia 56(9):1925–1933. https://doi.org/10.1007/s00125-013-2940-y
7. Mukamal KJ, Kizer JR, Djoussé L et al (2013) Prediction and classification of cardiovascular disease risk in older adults with diabetes. Diabetologia 56(2):275–283. https://doi.org/10.1007/s00125-012-2772-1
8. Conroy RM, Pyörälä K, Fitzgerald AP et al (2003) Estimation of ten-year risk of fatal cardiovascular disease in Europe: the SCORE project. Eur Heart J 24(11):987–1003. https://doi.org/10.1016/S0195-668X(03)00114-3
9. Vartiainen E, Laatikainen T, PeltonenM, Puska P (2016) Predicting coronary heart disease and stroke: the FINRISK calculator. Glob Heart 11(2):213–216. https://doi.org/10.1016/j.gheart.2016.04.007
10. Anderson KM, Odell PM, Wilson PW, Kannel WB (1991) Cardiovascular disease risk profiles. Am Heart J 121(1 Pt 2):293–298. https://doi.org/10.1016/0002-8703(91)90861-b
11. Wilson PWF, D’Agostino RB, Levy D, Belanger AM, Silbershatz H, Kannel WB (1998) Prediction of coronary heart disease using risk factor categories. Circulation 97(18):1837–1847. https://doi.org/10.1161/01.CIR.97.18.1837
12. Hippisley-Cox J, Coupland C, Vinogradova Y et al (2008) Predicting cardiovascular risk in England and Wales: prospective derivation and validation of QRISK2. BMJ 336(7659):1475–1482.
13. Hippisley-Cox J, Coupland C, Brindle P (2017) Development and validation of QRISK3 risk prediction algorithms to estimate future risk of cardiovascular disease: prospective cohort study. BMJ 357: j2099. https://doi.org/10.1136/bmj.j2099
14. Goff DC, Lloyd-Jones DM, Bennett G et al (2014) 2013 ACC/AHA guideline on the assessment of cardiovascular risk: a report of the American College of Cardiology/American Heart Association task force on practice guidelines. Circulation 129(25 Suppl 2):S49–S73. https://doi.org/10.1161/01.cir.0000437741.48606.98
15. Ridker PM, Paynter NP, Rifai N, Gaziano JM, Cook NR (2008) Creactive protein and parental history improve global cardiovascular risk prediction: the Reynolds risk score for men. Circulation 1 1 8 ( 2 2 ) : 2 2 4 3 – 2 2 5 1 . h t t p s : / / d o i . o r g / 1 0 . 1 1 6 1 /CIRCULATIONAHA.108.814251
16. Ridker PM, Buring JE, Rifai N, Cook NR (2007) Development and validation of improved algorithms for the assessment of global cardiovascular risk in women: the Reynolds risk score. JAMA 297(6):611–619. https://doi.org/10.1001/jama.297.6.611